In the rapidly evolving landscape of the insurance industry, predictive analytics is emerging as a game-changer. By leveraging vast amounts of data and sophisticated algorithms, insurers are now able to predict and assess risks with unprecedented accuracy, offering more tailored and affordable policies to customers.
Predictive analytics isn't a new concept, but its application in insurance has only recently begun to gain traction. Traditionally, insurers relied on historical data and static models to assess risk. However, these methods often failed to account for the dynamic nature of risk factors in today's world, leading to inadequate coverage or inflated premiums.
Enter predictive analytics—a paradigm shift that transforms how insurers operate. By tapping into big data sources such as social media, telematics, and IoT devices, insurers can now gather real-time information about clients' behaviors and lifestyles. This data is then processed through machine learning algorithms, allowing insurers to identify patterns and predict potential risks with remarkable precision.
One notable area where predictive analytics is making waves is in auto insurance. Telematics devices installed in vehicles collect data on driving habits, from speed and braking patterns to mileage and time of travel. Insurers can then use this information to create personalized premiums based on actual driving behavior rather than generic demographic factors. Safe drivers are rewarded with lower premiums, while risky drivers are incentivized to improve their habits.
Similarly, the health insurance sector is reaping the benefits of predictive analytics. Wearable devices, such as fitness trackers, monitor physical activity, heart rates, and sleep patterns. Insurers can use this data to offer customized wellness programs, promoting healthier lifestyles and reducing the likelihood of costly claims. Predictive models can also identify individuals at higher risk of chronic diseases, enabling proactive interventions and preventive care.
However, the adoption of predictive analytics in insurance is not without challenges. Privacy concerns and data security are major issues that insurers must address to gain the trust of customers. Transparent communication about data usage and robust security measures are critical to ensuring that clients feel confident in sharing their personal information.
Additionally, the accuracy and reliability of predictive models depend on the quality of data inputs. Insurers must invest in data management systems and ensure that the data they collect is clean, relevant, and up-to-date. They also need to continually refine their algorithms to adapt to changing trends and emerging risks.
Despite these challenges, the potential benefits of predictive analytics far outweigh the drawbacks. Insurers who embrace this technology stand to gain a competitive edge by offering more accurate risk assessments, fairer pricing, and enhanced customer experiences. As predictive analytics continues to evolve, it will undoubtedly reshape the insurance industry, paving the way for a more sophisticated and resilient future.
The rise of predictive analytics in insurance: transforming risk assessment
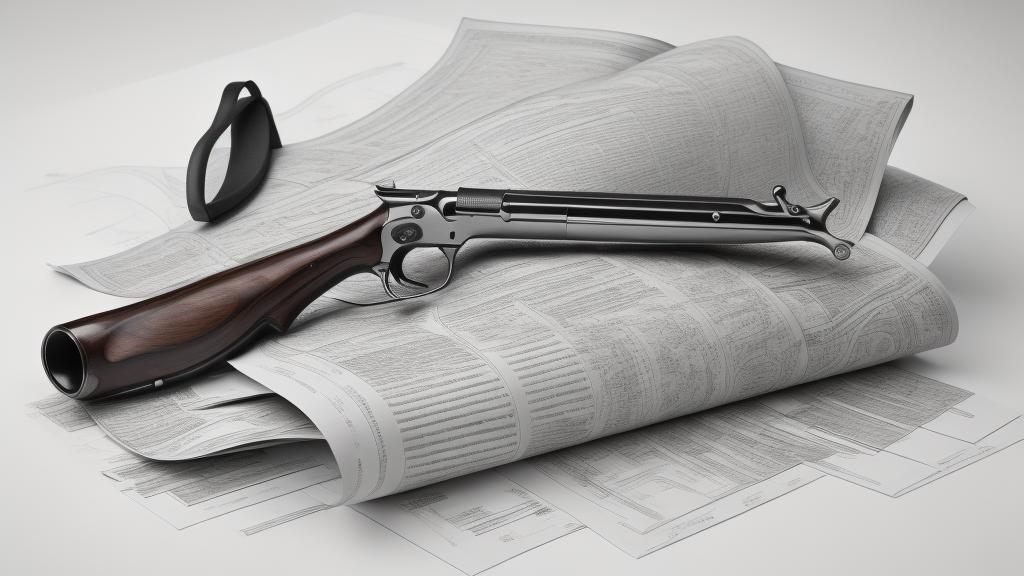